A Statistical Analysis of COVID-19 Image Detection Using the Wavelet Transform
Keywords:
Covid-19 detection,, Magnetism Resonant Imaging (MRI),, Calculated Tomography (CT),, Discrete Wavelet Transform (DWT),Abstract
Coronavirus (COVID-19), a newly discovered virus that shows similar symptoms to pneumonia, has been sweeping the world since December 2019. The World Health Organization (WHO) declared this disease a global pandemic because of its high contagiousness. This virus can cause fatal symptoms in some patients. Therefore, early detection of COVID-19 is crucial. The main challenge in detecting COVID-19 is that it affects the human body's respiratory systemTop of Form. In this work, wavelet transduction is used to integrate multifocal images in order to detect COVID-19. In order to detect COVID-19, MRI and CT were used. Clinical diagnoses were supported by the multifocal image. Seven wave-based algorithms were used: bior2.2, coif2, db2, dmey, rbio2.2, sym4, and haar. This method successfully integrates data acquired from CT and MRI scans, resulting in a merged image that enhances the efficiency of disease diagnosis. MATLAB is employed for evaluating the algorithm's effectiveness, with the entropy, PSNR and factor serving as metrics to assess the efficiency of image fusion. In a statistical analysis, the images demonstrated superior attributes over CT and MRI images.
Downloads
References
S. Udomhunsakul, and P. Wongsita, “Feature extraction in medical MRI images,“ Proceeding of 2004 IEEE Conference on Cybernetics and Intelligent Systems, vol. 1. p.p. 340- 344. 2004.
B. B. Hazarika, and D. Gupta "Modelling and forecasting of COVID-19 spread using wavelet-coupled random vector functional link networks", Applied Soft Computing, vol. 96, p. 106626, 2020.
M. Pahar, M. Klopper, R. Warren, and T. Niesler, "COVID-19 detection in cough, breath and speech using deep transfer learning and bottleneck features", Computers in biology and medicine, vol. 141, p. 105153, 2022.
V. Despotovic, M. Ismael, M. Cornil, R. Mc Call, & G. Fagherazzi, "Detection of COVID-19 from voice, cough and breathing patterns: Dataset and preliminary results", Computers in Biology and Medicine, vol. 138, p. 104944, 2021.
Y. E. Erdoğan, & A. Narin, "COVID-19 detection with traditional and deep features on cough acoustic signals", Computers in Biology and Medicine, vol. 136, p. 104765, 2021.
A. Sarhan. "Run length encoding based wavelet features for COVID-19 detection in X-rays". BJR| Open, vol. 3. No. 1. p. 20200028, 2021.
K. Parmar, K. K. Rahul, and N. T. Falgun, "Analysis of CT and MRI on image fusion using wavelet transform." 2012 International Conference Communication Systems and Network Technologies. IEEE 2012.
S. Mane, and S. D. Sawant, "Image Fusion On Mr And Ct Images Using Wavelet Transforms And Dsp Processor." International Journal of Engineering Trends and Technology (IJETT), vol. 4, 2013.
C. Y. N. Dwith, V. Angoth and A. Singh, "Wavelet based image fusion for detection of brain tumor", International Journal of Image, Graphics and Signal Processing, vol. 5, no. 1, p.p. 25, 2013.
R. J. Sapkal, and S. M. Kulkarni, "Image fusion based on wavelet transform formedical application", International Journal of Engineering Research and Applications, vol.2.5, p.p. 624-627, 2012.
I. Powell, M. J. D. "An algorithm for maximizing entropy subject to simple bounds", Mathematical Programming, vol. 42, no. (1), p.p. 171-180, 1988.
Wang Zhou, Bovik, Alan C., Sheikh, Hamid R., and Simoncelli, Eero P.Image Quality Assessment: From Error Visibility to Structural Similarity. IEEE Transactions on Image Processing, vol.13, Issue 4, pp. 600–612, April 2004.
Zhou Wang and Alan C. Bovik, “A Universal Image Quality Index,” IEEE Signal Processing Letters,vol. 9, no. 3, pp. 81-84, Mar. 2002
D. Niranjan et al. “Image Quality Assessment Based on a Degradation Model,” IEEE Transaction on Image Processing, vol. 9, NO. 4, April 2000
ITU, “Methods for the Subjective Assessment of the Quality of Television Pictures,” August 1998, ITU-R Rec. BT. 500-7.
X. Otazu, C. Parraga, and M. Vanrell, “Toward a unified chromatic induction model,” Journal of Vision, vol. 10(12), no. 6, 2010.
Gonzalez and Woods, “Digital Image Processing using MATLAB”, Prentice Hall, New Delhi, India, Year 2006.
Alan C. Brooks, Xiaonan Zhao, and Thrasyvoulos N. Pappas, “Structural Similarity Quality Metrics in a Coding Context: Exploring the Space of Realistic Distortions”, IEEE Transactions on image processing, vol. 17, No. 8, August 2008.
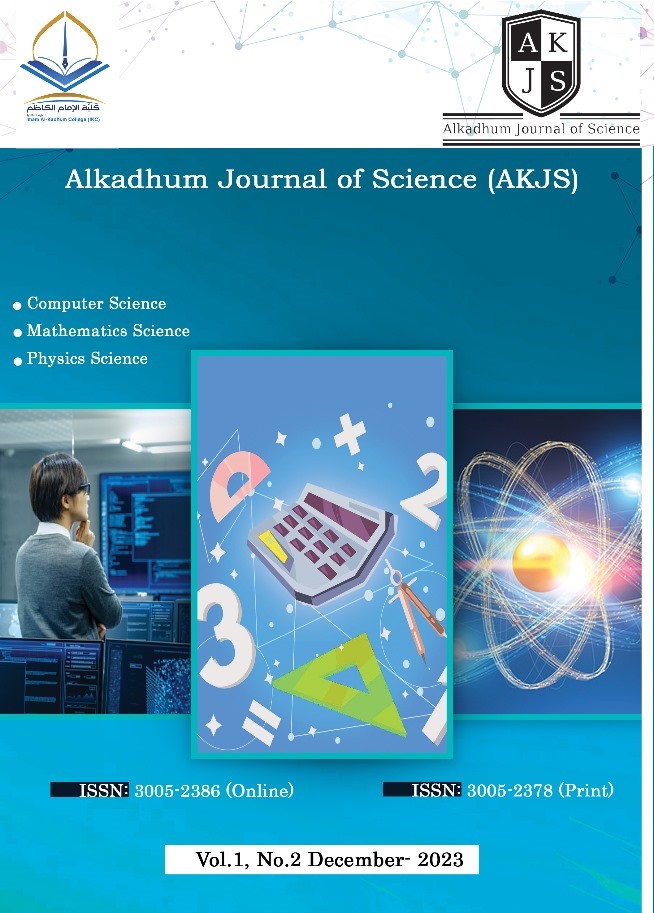